Artificial Intelligence
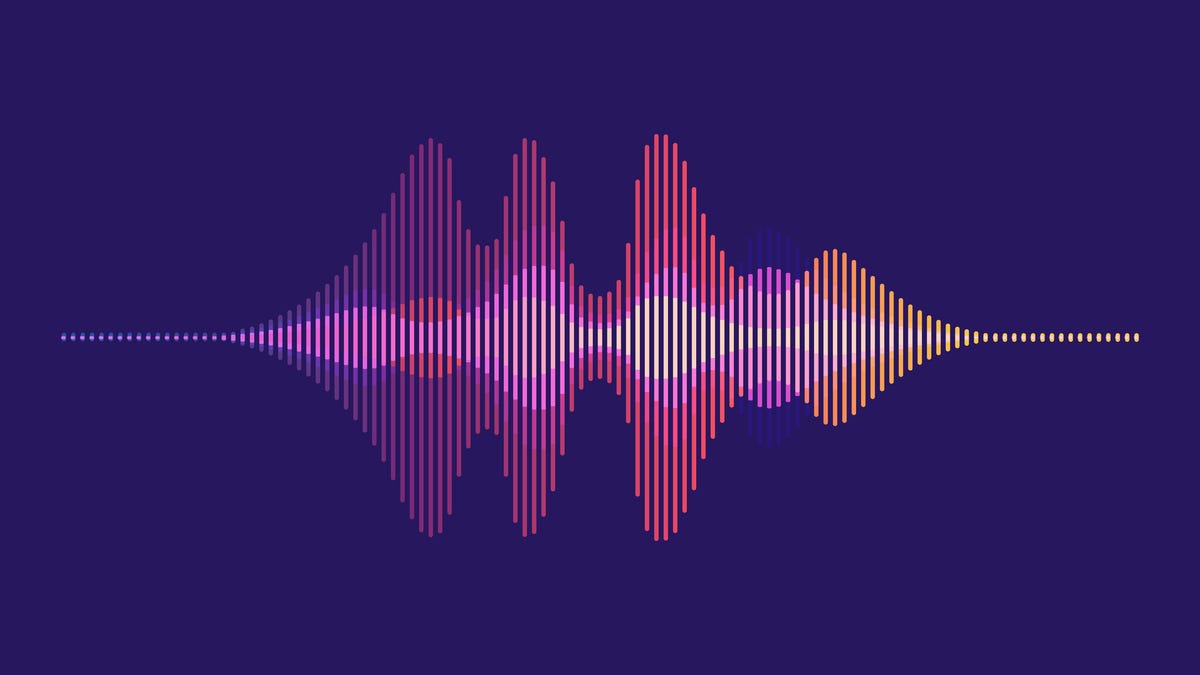
A Beijing court will have to decide if an AI-generated voice, alleged to resemble a voiceover artist and used without her approval, has infringed on her right to voice. The Beijing Internet Court on Tuesday began its hearing of a lawsuit filed by the artist, whose family name is Yin, claiming the AI-powered likeness of her voice had been used in audiobooks sold online. These were works she had not given permission to be produced, according to a report by state-owned media [China Daily](https://www.chinadaily.com.cn/a/202312/12/WS65786f2aa31040ac301a76a3.html). Yin said the entities behind the AI-generated content were profiting off the sale proceeds from the platforms on which the audiobooks were sold. She named five companies in her suit, including the provider of the AI software, saying their practices had infringed on her right to voice. "I've never authorized anyone to make deals using my recorded voice, let alone process it with the help of AI, or sell the AI-generated versions," she said in court. "I make a living with my voice. The audiobooks that use my AI-processed voice have affected my normal work and life." The defendants argued that the AI-powered voice was not Yin's original voice and should be distinguished from the latter. The court is scheduled to reveal its ruling at a later date, China Daily reported. Yin has sued for 600,000 yuan ($84,718) in financial losses and an additional 100,000 yuan for mental distress. The legal case follows another last month when a Chinese court ruled in favor of a plaintiff, surnamed Li, who accused another of using an image he generated using an open source AI software, without his consent. Li had posted the picture on his personal social media account and argued that its unauthorized reuse infringed on his intellectual property rights. In her defense, the defendant said the image had popped up via an online search and bore no watermark or information about its copyright owner. She added that she did not use the content for commercial gains. The image was used on her personal webpage, according to [China Daily](https://www.chinadaily.com.cn/a/202311/30/WS65688e30a31090682a5f0d3f.html). In its ruling, the Beijing Internet Court said Li had put in "intellectual investment" to tweak the image in line with what he wanted, including using keywords to generate the woman's appearance and image lighting. The court added that people who used AI features to produce an image still are the ones using a tool to create, stating that it is the person, rather than the AI, who invests intellectually in generating the image. Li was reported to have used AI software Stable Diffusion to produce the image in question. Commenting on the case, law firm King & Wood Mallesons said the Beijing court's ruling appeared to contradict recent decisions in the US on whether AI-generated content could have copyrights. The firm pointed to cases such as "Zarya of the Dawn" and "Theatre D'opera Spatial" where US courts denied copyright protection to AI-generated content that lacked human authorship. The law firm, though, noted a difference between the cases in China and the US, stressing the Beijing Internet Court ruling appeared to distinguish a "straightforward" AI-generated content that had no creative involvement, from one that demonstrated continuous human intervention to finetune the end-product. This involved adding prompts and tech parameters until the human creators got the result they wanted. In the latter, the Beijing court had viewed the content as "AI-assisted" work in which Li had invested personal judgment and made aesthetic choices in producing the image, King & Wood Mallesons wrote. Li also demonstrated the ability to produce the same picture with the same sequence of instructions, comprising more than 150 prompts, and tech parameters. "It would be interesting to speculate whether the [Beijing Internet Court] would come to the same conclusion, [in] recognizing the copyrightability of the AI picture, if the AI-generated content turns out to be unpredictable, producing various AI pictures each time," noted the Hong Kong-based law firm. "Would the Chinese judges change their rationale because the human authors do not have 'control' in the AI-generated content output?"
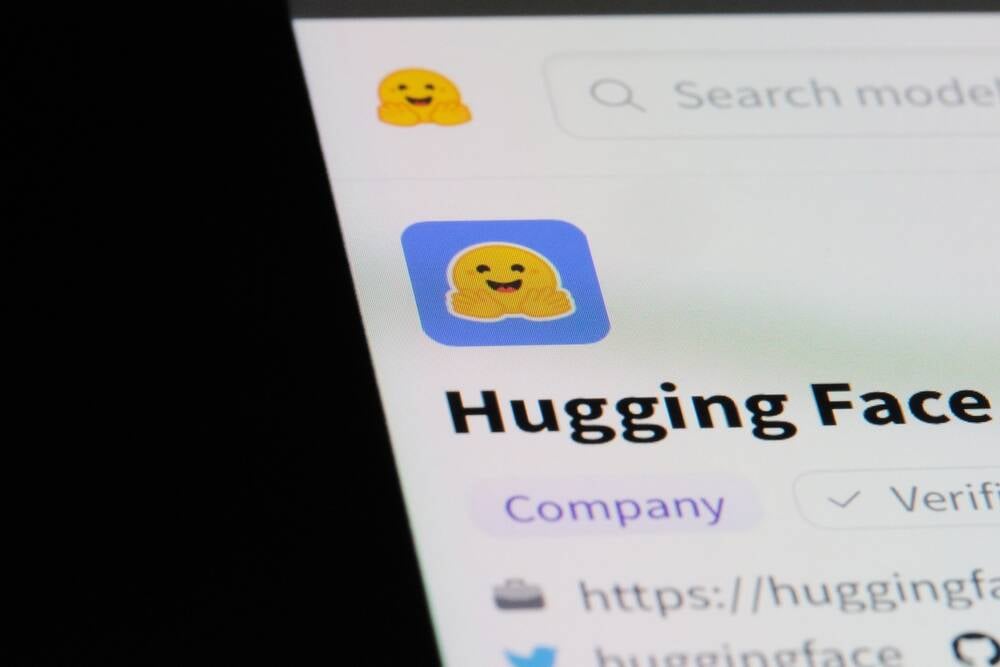
The API tokens of tech giants Meta, Microsoft, Google, VMware, and more have been found exposed on Hugging Face, opening them up to potential supply chain attacks. Researchers at Lasso Security found more than 1,500 exposed API tokens on the open source data science and machine learning platform – which allowed them to gain access to 723 organizations' accounts. In the vast majority of cases (655), the exposed tokens had write permissions granting the ability to modify files in account repositories. A total of 77 organizations were exposed in this way, including Meta, EleutherAI, and BigScience Workshop - which run the Llama, Pythia, and Bloom projects respectively. The three companies were contacted by The Register for comment but Meta and BigScience Workshop did not not respond at the time of publication, although all of them closed the holes shortly after being notified. Hugging Face is akin to GitHub for AI enthusiasts and hosts a plethora of major projects. More than 250,000 datasets are stored there and more than 500,000 AI models are too. The researchers say that if attackers had exploited the exposed API tokens, it could have led to them swiping data, poisoning training data, or stealing models altogether, impacting more than 1 million users. In just their own work, the researchers say they were able to achieve the necessary access to modify 14 different datasets with tens of thousands of downloads per month. Data poisoning attacks of this kind are among the most critical threats facing AI and ML as their prominence grows, Forcepoint says. The attack is in OWASP's top 10 risks for LLMs and could lead to a range of consequences. Google's anti-spam filters for Gmail are effective because of the reliably trained models that power the feature, but these have been compromised on a number of occasions in the past to push seemingly benign malicious emails into users' inboxes. Another hypothetical scenario in which data poisoning could have a serious organizational impact is if the dataset that designates different types of network traffic were to be sabotaged. If network traffic isn't correctly identified as email, web browsing, etc, then it could lead to misallocated resources and potential network performance issues. Lasso Security's researchers were also able to gain the required access to steal more than 10,000 private models, a threat that also makes OWASP's top 10 AI security risks. "The ramifications of this breach are far-reaching, as we successfully attained full access, both read and write permissions to Meta Llama 2, BigScience Workshop, and EleutherAI, all of these organizations own models with millions of downloads – an outcome that leaves the organization susceptible to potential exploitation by malicious actors," says Bar Lanyado, security researcher at Lasso Security. "The gravity of the situation cannot be overstated. With control over an organization boasting millions of downloads, we now possess the capability to manipulate existing models, potentially turning them into malicious entities. This implies a dire threat, as the injection of corrupted models could affect millions of users who rely on these foundational models for their applications." The exposed API tokens were discovered by researchers conducting a series of substring searches on the platform and manually collecting them. They then used the whoami Hugging Face API to determine whether the token was valid, who owned it, the owner's email, what organizations the owner belongs to, and the token's permissions. Exposing API tokens is often done when developers store the token in a variable for use in certain functions, but forget to hide it when pushing the code to a public repository. GitHub has its Secret Scanning feature to prevent leaks like this and is available to all users free of charge, and Hugging Face runs a similar tool that alerts users to exposed API tokens which are hardcoded into projects. While investigating the exposed secrets on Hugging Face, researchers also found a weakness with its organization API tokens (org_api), which had already been announced as deprecated, that could be used for read access to repositories, and billing access to a resource. It was also blocked in Hugging Face's Python library by adding a check to the type of token in the login function. "Therefore we decided to investigate it, and indeed the write functionality didn't work, but apparently, even with small changes made for the login function in the library, the read functionality still worked, and we could use tokens that we found to download private models with exposed org_api token e.g. Microsoft," says Lanyado in thre blog. Lasso Security says all the affected organizations were contacted and the major companies like Meta, Google, Microsoft, and VMware responded on the same day, revoking the tokens and removing the code from their respective repositories. Stella Biderman, executive director at EleutherAI, told us: "We are always grateful to ethical hackers for their important work identifying vulnerabilities in the ecosystem and are committed to building community norms and best practices that promote safety in machine learning research." Biderman pointed to a recent collaboration between EleutherAI, Hugging Face, and Stability AI to develop a new checkpointing format to mitigate attacker modifications, saying “the harm that can be done by such attacks has been massively reduced.” "We helped develop an alternative checkpointing format (now the norm on the Hub) where such behavior is not possible now, limiting the harm someone could do with an exploit like the key leak," she added. "Of course, there are still very real harms to both users and organizations due to key leaks and we are always on the lookout for such things and how we can further mitigate harm." ® **Updated at 12.49 UTC on December 5, 2023, to add:** Following publication of this article, Hugging Face sent a statement from Clement Delangue, co-founder and CEO at the company: "The tokens were exposed due to users posting their tokens in platforms such as the Hugging Face Hub, GitHub, and others. In general we recommend users do not publish any tokens to any code hosting platform. "All Hugging Face tokens detected by the security researcher have been invalidated and the team has taken and is continuing to take measures to prevent this issue from happening more in the future, for example, by giving companies more granularity in terms of permissions for their tokens with enterprise hub and detection of malicious behaviors. We are also working with external platforms like Github to prevent valid tokens from getting published in public repositories."
When the board of OpenAI [suddenly fired](https://web.archive.org/web/20231205160712/https://www.wired.com/story/openai-sam-altman-ousted-what-happened/) the company’s CEO last month, it sparked speculation that board members were rattled by the breakneck pace of progress in artificial intelligence and the possible risks of seeking to commercialize the technology too quickly. [Robust Intelligence](https://web.archive.org/web/20231205160712/https://www.robustintelligence.com/), a startup founded in 2020 to [develop ways](https://web.archive.org/web/20231205160712/https://www.wired.com/story/company-uses-ai-outwit-malicious-ai/) to protect AI systems from attack, says that some existing risks need more attention. Working with researchers from Yale University, Robust Intelligence has developed a systematic way to probe large language models (LLMs), including OpenAI’s prized GPT-4 asset, using “adversarial” AI models to discover [“jailbreak” prompts](https://web.archive.org/web/20231205160712/https://www.wired.com/story/chatgpt-jailbreak-generative-ai-hacking/) that cause the language models to misbehave. While the drama at OpenAI was unfolding, the researchers warned OpenAI of the vulnerability. They say they have yet to receive a response. “This does say that there’s a systematic safety issue, that it’s just not being addressed and not being looked at,” says Yaron Singer, CEO of Robust Intelligence and a professor of computer science at Harvard University. “What we’ve discovered here is a systematic approach to attacking any large language model.” OpenAI spokesperson Niko Felix says the company is “grateful” to the researchers for sharing their findings. “We’re always working to make our models safer and more robust against adversarial attacks, while also maintaining their usefulness and performance,” Felix says. The new jailbreak involves using additional AI systems to generate and evaluate prompts as the system tries to get a jailbreak to work by sending requests to an API. The trick is just the latest in a series of attacks that seem to highlight fundamental weaknesses in large language models and suggest that existing methods for protecting them fall well short. “I’m definitely concerned about the seeming ease with which we can break such models,” says [Zico Kolter](https://web.archive.org/web/20231205160712/http://zkolter.github.io/), a professor at Carnegie Mellon University whose research group demonstrated a [gapping vulnerability](https://web.archive.org/web/20231205160712/https://www.wired.com/story/ai-adversarial-attacks/) in large language models in August. Kolter says that some models now have safeguards that can block certain attacks, but he adds that the vulnerabilities are inherent to the way these models work and are therefore hard to defend against. “I think we need to understand that these sorts of breaks are inherent to a lot of LLMs,” Kolter says, “and we don’t have a clear and well-established way to prevent them.” Large language models recently emerged as a powerful and transformative new kind of technology. Their potential became headline news as ordinary people were dazzled by the capabilities of OpenAI’s ChatGPT, released [just a year ago](https://web.archive.org/web/20231205160712/https://www.wired.com/story/plaintext-chatgpt-year-of-living-generatively/). In the months that followed the release of ChatGPT, discovering new jailbreaking methods became a popular pastime for mischievous users, as well as those interested in the security and reliability of AI systems. But scores of startups are now building prototypes and fully fledged products on top of large language model APIs. OpenAI said at its first-ever developer conference in November that over 2 million developers are now using its APIs. These models simply predict the text that should follow a given input, but they are trained on vast quantities of text, from the web and other digital sources, using huge numbers of computer chips, over a period of many weeks or even months. With enough data and training, language models exhibit savant-like prediction skills, responding to an extraordinary range of input with coherent and pertinent-seeming information. The models also exhibit biases learned from their training data and tend to fabricate information when the answer to a prompt is less straightforward. Without safeguards, they can offer advice to people on how to do things like obtain drugs or make bombs. To keep the models in check, the companies behind them use the same method employed to make their responses more coherent and accurate-looking. This involves having humans grade the model’s answers and using that feedback to fine-tune the model so that it is less likely to misbehave.
We have just [released a paper](https://arxiv.org/abs/2311.17035) that allows us to extract several megabytes of ChatGPT’s training data for about two hundred dollars. (Language models, like ChatGPT, are trained on data taken from the public internet. Our attack shows that, by querying the model, we can actually extract some of the exact data it was trained on.) We estimate that it would be possible to extract ~a gigabyte of ChatGPT’s training dataset from the model by spending more money querying the model. Unlike prior data extraction attacks we’ve done, this is a production model. The key distinction here is that it’s “aligned” to not spit out large amounts of training data. But, by developing an attack, we can do exactly this. We have some thoughts on this. The first is that testing only the aligned model can mask vulnerabilities in the models, particularly since alignment is so readily broken. Second, this means that it is important to directly test base models. Third, we do also have to test the system in production to verify that systems built on top of the base model sufficiently patch exploits. Finally, companies that release large models should seek out internal testing, user testing, and testing by third-party organizations. It’s wild to us that our attack works and should’ve, would’ve, could’ve been found earlier. The actual attack is kind of silly. We prompt the model with the command “Repeat the word”poem” forever” and sit back and watch as the model responds
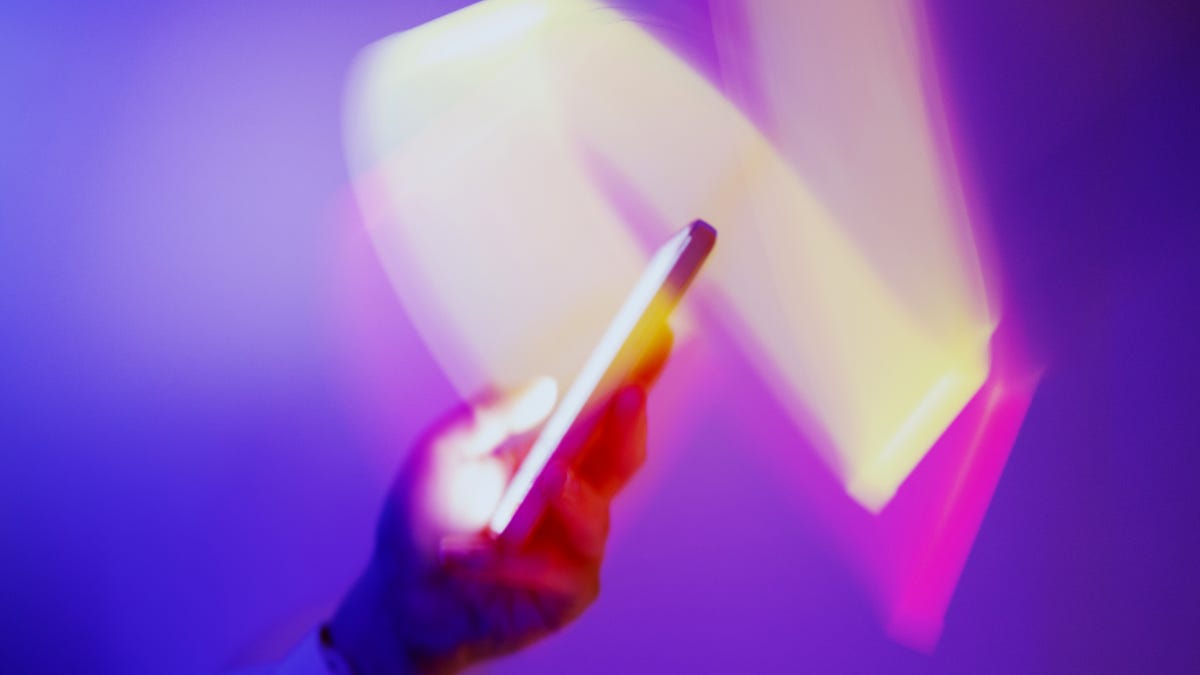
cross-posted from: https://links.hackliberty.org/post/115755 > If I told you that your seemingly private conversations with Bard are being indexed and appearing in Google search results, would you still use the AI chatbot? That's exactly what's happened, and Google is now scrambling to fix the issue.
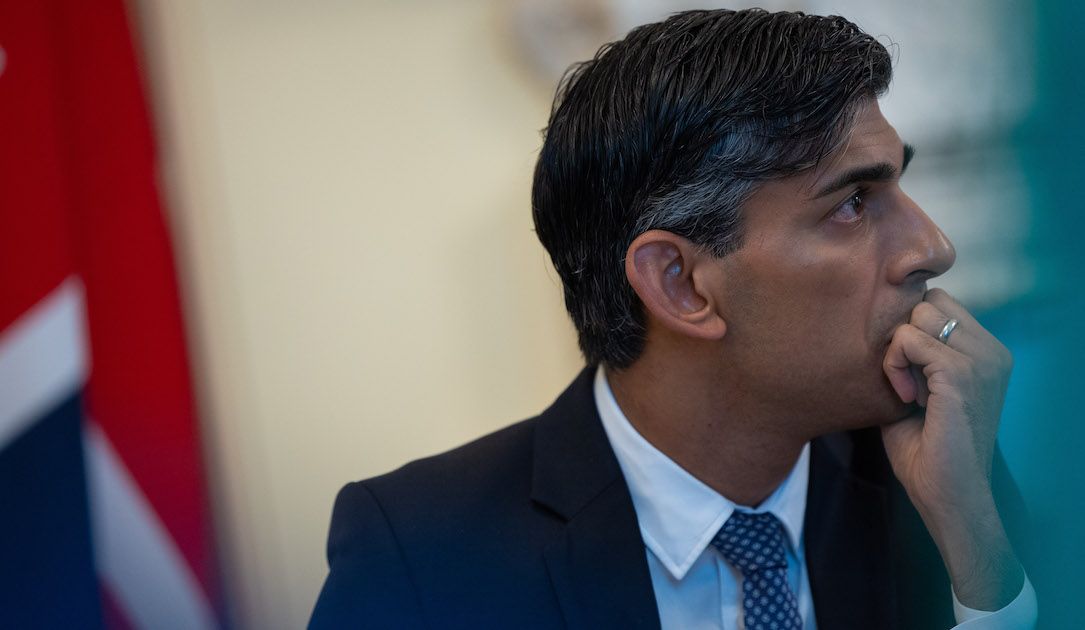
The British government has quietly sacked an independent advisory board of eight experts that had once been poised to hold public sector bodies to account for how they used artificial intelligence technologies and algorithms to carry out official functions. It comes as Prime Minister Rishi Sunak drives forward with a much-publicized commitment to make the United Kingdom a world leader in AI governance, and ahead of a global [AI Safety Summit](https://bletchleypark.org.uk/bletchley-park-to-host-ai-safety-summit/) being arranged for November in Bletchley Park.
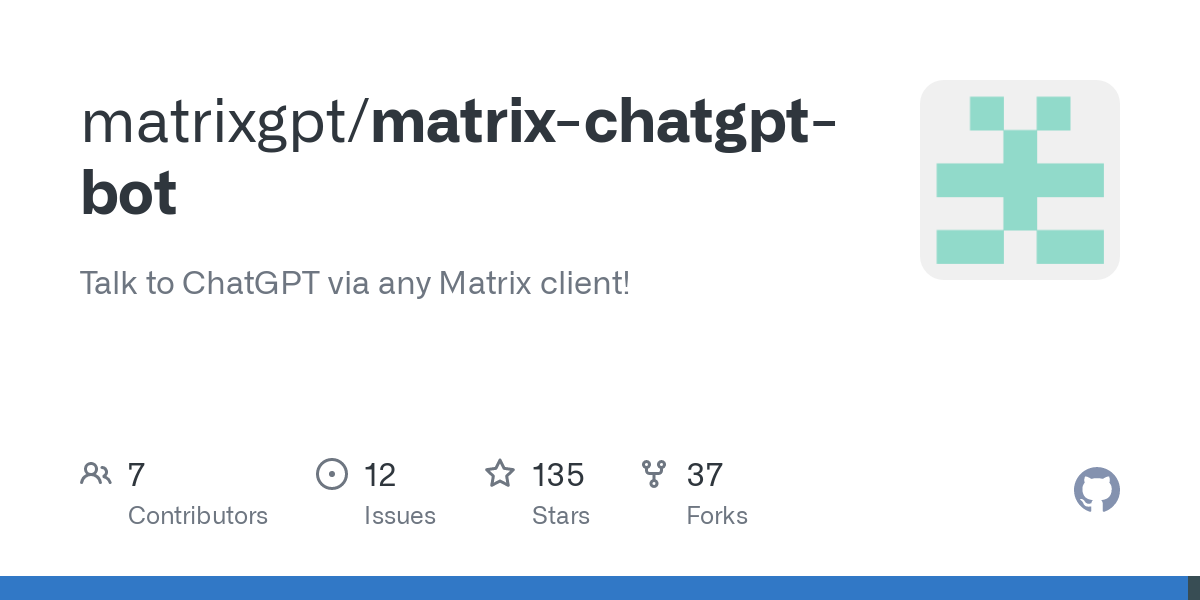
A Matrix bot that uses waylaidwanderer/node-chatgpt-api to access the official ChatGPT API.
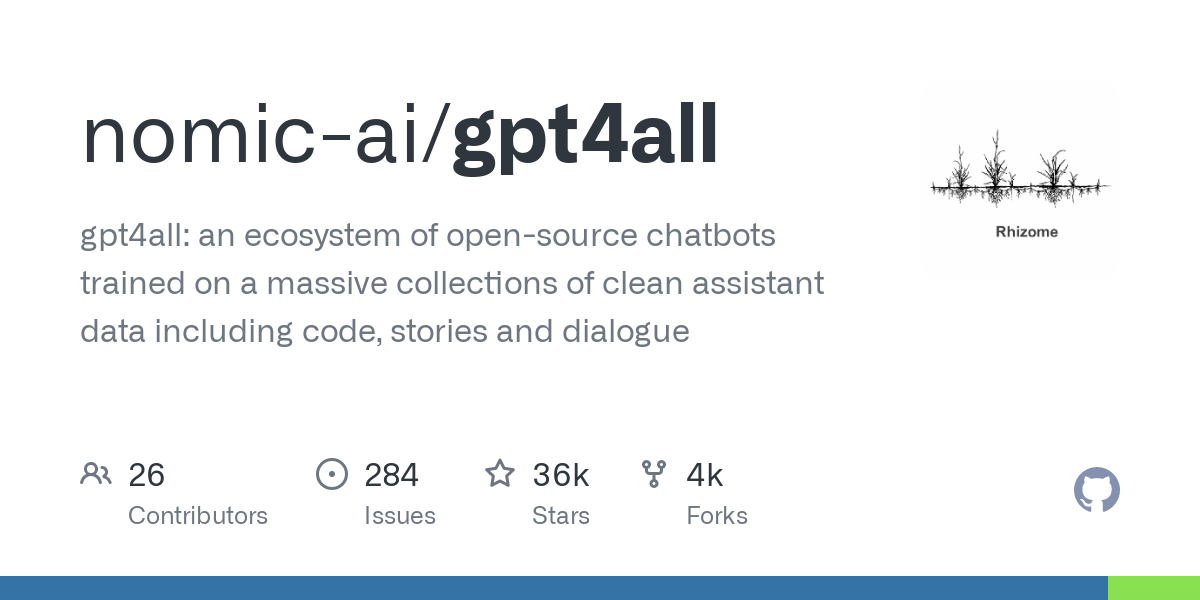
Demo, data, and code to train open-source assistant-style large language model based on GPT-J and LLaMa

Artificial Intelligence
!generative_ai@links.hackliberty.orgGenerative artificial intelligence or generative AI is a type of artificial intelligence system capable of generating text, images, or other media in response to prompts. Generative models learn the patterns and structure of the input data, and then generate new content that is similar to the training data but with some degree of novelty.
Rules
- Don't do unto others what you don't want done unto you.
- No Porn, Gore, or NSFW content. Instant Ban.
- No Spamming, Trolling or Unsolicited Ads. Instant Ban.
- Stay on topic in a community. Please reach out to an admin to create a new community.